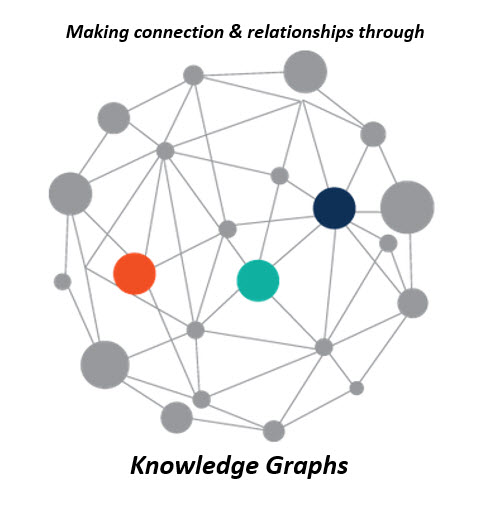
Knowledge Graphs have a real potential to become highly valuable, topical and relevant. If only we can get them prised out of the engineer, data scientists, or software experts hands.
We simply should so we can get this concept fully out into the real world, that of applying as solutions to real client problems, it would really help. I get tired of hearing about “use cases”, where concepts like KG often get caught up in, that never-ending validation.
Is this validation simply because it does not work, it is too much hard work delivering the promise within the concept? Or the approach has too much complexity around it and needs massive resources to undertake?
KG needs a real resource momentum and a determination to break through uncertainty. Its huge value should drive it, and caution should be modified and lets go out and validate it, in the real world.
If any of these “constraints” are the case, then we do need to “hack this” differently, as Knowledge Graphs has what I see an incredible potential, as an application solution that should be deemed as far too important to keep under wraps. We need to instill a sense of urgency into this. Why, well read on.
Here I want to give a deeper explanation of knowledge graphs- a ‘potted’ history and future view. So, my attempt here is to give Knowledge Graphs a more ”layman” context so we can begin to see how this “Knowledge Graph” concept can become really important to recognize, support and apply in our lives.
It will equally become a significant industrial application as well, as increasingly be part of our everyday working life in related searches as it is today by our personal use of Google or other search engines that have Knowledge Graphs increasingly sitting behind them. It might become the next big “buzz”, I recently heard being as topical as “Artifical Intelligence” as a prediction for use in an industrial application. As big a buzz- wow.
It is once we make all the connects in the knowledge that is out there, waiting to form new relationships, we unleash our connected understanding in completely new ways.
Knowledge Graphs help us to form essential context and that has been often missing or simply the poor cousin to content. Context is king, not content!
Struggling to get out of the technical jargon, so be ready and have patience.
Most of what I have read and researched gives detailed explanations of how they work and throws around all the different jargon, protocols and such, as the need to understand the important semantic technologies, concepts, and methods like RDF, URI, SPARQL, SKOS, and OWL. That is fine but we need to turn Knowledge Graphs into the compelling story I think it is but it always have to be provided in layman’s terms, otherwise, it becomes a great idea that struggles to get its importance across, to those that are the eventual decision makers, or those that have to make it work.
As we all know, the biggest buzz on the block today is “Artificial Intelligence”, well it is within this Knowledge Graphs we have a large part of its foundation. AI is getting to a point where it will drive the next wave of technology disruption. Building AI application requires Context. Knowledge Graphs provide the context.
If we don’t have increasing context we will never realize our existing human or artificial intelligence as it constantly fails to connect-up all the parts, in any search or relating knowledge. We see the Web as increasingly the point of knowledge or referral point. That works to a point but all our internal organization data is not on the web, it still sits in silos.
Google, a leader in AI introduced in 2012 “Knowledge Graphs”. As I have heard it explained they moved from links or strings to turn them more into “things”. This now allows us to have far more multiple options and it is the graph database behind this that is providing the structure, relationships and detailed information about the topic you are wanting to find out more. It is providing more options and choices of where to go in your next step, partly as it has been set up to connect to search more on “things” or concepts.
I wanted to put in here Googles view of a layman’s guide, which I can only dare you to explore. It left me feeling totally inadequate as a real layman. Go on, beat yourself up on this but come back here as this is a real layman’s guide.
Knowledge Graphs capture relationships between people, processes, applications, data, things and their relationships. The more the connections are connected the more context you can be provided to make more informed choices. Knowledge Graphs feed off more related data to give increasing contextual information.
Machine learning works at understanding dependencies so emerging from any learning you get a growing sense of intent, objective and those important aspects that you are wanting to find out more upon, to build out even further. The move from just “stringing data together” to build a picture of ‘things’ you get greater contextual knowledge.
Today we have always struggled with where our knowledge lies
It often stays ‘resident’ with the worker, scientist or locked into more traditional enterprise applications, there for one job but not recognized as connected to other jobs you might want to do. The querying of data still has limitations without significant rewriting of new code to go and undertake a search. We need to synthesize, amalgamate, classify, coordinate, organize data, information, and knowledge in multiple “different” ways to look deeper into alternatives and new choices. Our decisions are becoming more reliant on complexity and we need to organize our data differently to manage this. Knowledge Graphs provide part of the solution.
Within my researching around Knowledge Graphs, I came across the approach by Thomson Reuters and their Knowledge Graph feed is where they are applying key identifiers to make the connections you might miss by not having a structured Metadata that builds a richer content. I think this visual (shown below) of theirs helps begin to frame where Knowledge Graphs can go for building related documents to extract, classify and tag metadata. Metadata is data about data. It describes the underlying characteristics of the data by keywords describing the content and structural information. Metadata is critical to building Knowledge Graphs.
Go to Thompson Reuters intelligent tagging page where they outline how turns large amounts of unstructured data can become into a precise advantage, to tag the people, places, facts, and events in your content to increase its value, accessibility, and interoperability.

We are in need of building semantic relationships constantly for today’s connected world.
For example, multiple documents have within them that critical semantic relationship between the keywords, phrases, and concepts. As we can group these the relationships can potentially expose new information about their interrelationship, you might not have seen or been aware of, without making that critical connecting up. We can form deeper meaning or even gain new meaning, besides gaining a more comprehensive meaning, from forming this relationship.
The semantic knowledge graphs become the backbone of a new architecture that can enable entity-centric (things) to offer views on information, data, products, suppliers, employees, locations and research topics. Knowledge can be retrieved between these various connected objects and they convert previously unorganized data into useful informing information to determine a new action, or insight, or awareness.
The world-wide-web needs different machine learning methods and the application of new algorithms is helping knowledge graphing to catch up. We see Microsoft, Google, Amazon, along with a host of other “search” providers are all working on bringing semantic knowledge graphs to us. Knowledge Graphing has been transforming our searches on the web, now it needs to transform our daily lives, inside organizations and for us personally. Go check out Googles Knowledge Graphs.
Late last year (November 2017) Amazon announced its Neptune a fully-managed graph database service with the objective of building and running applications that work with highly connected data sets. This database engine is optimizing and storing billions of relationships and querying the graph with millisecond latency. It is offered in the cloud, very scalable and works for open graph API’s.
My interest spiked last December with a visit to Siemens R&D center
I wrote a post “As we enter 2018 we will need Knowledge Graphs” in December 2017, from that brief visit to Siemens where I saw an early conceptual, prototype, Knowledge Graph demonstration. It turned a light bulb on for me and I saw the future of “connected innovation”. Everything we all do is based on bringing data, information and turning this into knowledge. It is connecting this all up we have the greater potential for seeing new knowledge possibilities. We begin to question current or existing knowledge as we gain greater connections and new innovation is often born from this.
Grasping the industrial application of Knowledge Graphs
The industrial application of Knowledge Graphs will build the significant “domains of meaning” that is presently isolated in silo’s, caught up in single ownership, or trapped in known locations like R&D, Production, Marketing that are not linked. Then you have the actual media that is “trapped” in PDF, word, manuals, guidelines, all operating in islands of understanding. Without a common thread of data accessibility, we suffer from a lack of no integrated view, we limit our search functions and we continue to build inefficient workflows.
It is this lack of connecting all of this up really is placing demands on organizations that manage knowledge today, often very inefficiently. They constantly are turning to limited experts who often hold a selected knowledge in their head. Yet often these “experts” fail to see the “rich” possibilities in connecting-up all aspects of knowledge, as they simply do not have all the experiences and practical understanding of the very different connections and possibilities. Domain experts do.
The promise of Knowledge Graphs allows for the collected data and the connections to provide insight, influence decisions and set direction. The more you “see” all the connections and relationships the better these become.
Nearly every enterprise has a huge amount of data hidden in textual form (documents and files) and it is estimated 90% of this enterprise data is unstructured but if properly organized, it could create better customer experience, greater efficiencies, bring better products based on increased insight to market and constantly look to reduce inefficiencies and costs across the enterprise value chain.
We are told Artificial Intelligence is meant to be a panacea to solve all this current ‘disconnects’. No, not without solutions like Knowledge Graphs alongside them, well actually underpinning AI. As we digitally transform and improve the IoT through cognitive/ AI solutions we will automate and personalize communications. It is recognizing the semantic technologies part that will deliver cognitive solutions that have the real value.
This value can be built in very different ways than today. It can have the incredible value of being driven by domain experts and actual business users, as they make and use their knowledge to make increased the connections, not an IT professional, unfamiliar on the relationships or lacking deep domain knowledge. That’s part of the promise of Knowledge Graphs, built by those that know, not by ones who think they know! Understanding relationships are down often to the experience you have in the domain of that knowledge
Domain experts are the new influencers of tomorrow, not media influencers as we see today. Content needs context.
As I have outlined early Knowledge Graphs underpin Artificial as well as Human Intelligence.
It is the ‘enriching of data’ by building descriptive aspects, forming and applying context, building solutions that are interoperative, able to be queried and searched that can give a very different granularity.
As we build concepts (things, again) these are units of meaning that can then link to other concepts, thus creating contextual frameworks that open-up to new possibilities. Context informs us of richer meaning. It is the building of conceptual networks both in us as humans and in our learning capabilities of computer systems give us fresh knowledge bases.
In the past, we have attempted to create taxonomies and ontologies with one arm tied behind our back. They were excessive in resources to build but with the understanding of building these through Knowledge Graphs and being increasing enhanced by machine-learning algorithms, coupled with software solutions specific to the knowledge building task, we are getting closer to knowledge-driven reasoning, based on having a wider, connected understanding.
The ability to have greater intelligence at your fingertips holds the promise of improved ways to find, reuse, reconfigure and adapt relevant information. It can form the basis of the creation phase, providing suggestions in connections for new content.
So Knowledge Graphs build the domain knowledge by making explicit the concepts and relationships of entities (things). They are the backbone of cognitive and semantic solutions. They ‘advance search, personalization, and recommendations, based on greater connected knowledge. They turn unstructured data into structural ones that offer more connected capability.
Let’s return to some basic stuff to know about how Knowledge Graphs connect.
This is if you have not read Googles laymen’s guide mentioned above- without going into the real nitty-gritty details of Knowledge Graphs.
As brief an understanding as I, as a layman can give. We are wanting to link data. Something I still have to get my head around is RDF’s – So far I think these are binary relationships (subject, predicate, and object, SPO’s) where subject and object are entities and a predicate is a relationship between the two. So are you with me so far?
Drawing from one paper I read “A Review of Relational Machine Learning for Knowledge Graphs, written by Messrs Nickel, Murphy, Tresp, and Gabrilovich in 2015, that gave a good example as shown above and the emerging relationships below.
It is the existence of a triple SPO indicates an existing fact. It is when we combine multiple SPO’s we form multiple graphs. Here it is nodes that represent entities (all subjects and objects) and directed edges represent relationships and where they ‘point’ builds the knowledge graph. Each SPO is a (stand-alone) fact. So we begin to build a Knowledge Graph as the above example shows.
Let me give you an outline of benefits of why Knowledge Graphs – seen by Siemens
This might help lift the understanding around Knowledge Graphs. Why Knowledge Graphs can become the “next big thing” to understand and relate too for industrial application. You connect people and their knowledge in different domains, the knowledge sharing, and the platform sharing. The value builds from greater connections and relationships.
Then the way Siemens see knowledge – as a service – that works within their Mindsphere platform
The feeding in of understanding, into any platform like Mindsphere, improves perception, reasoning, and decision-making. You get a more “intelligible domain model” of something that might be complex, that many people (partners) need to understand and can increasingly relate too. More people connect and build deeper relationships with shared knowledge. More on this in a further post, sometime in the near future.
Why I see Knowledge Graphs as important
For industrial applications why do I see these Knowledge Graphs as important? They help in decision potential, in decision making, influencing to improve collective knowledge or signaling a greater potential can all be greater in possibility. Now that has the real power of combining knowledge graphs, extracted from related data, applying learning algorithms to end up with the outcome of Augmented Intelligence. This KG approach has the potential of being the knowledge that can lead to unlocking problems, and exploring new potential in design, solutions or prompting new actions.
If I have not lost you at this point, Knowledge Graphs move us from those islands of data, held in silo’s, we can begin to build through knowledge digitalization domain-specific knowledge, and through the building and increasing these through automated structures we can begin to discover new understanding behind knowledge as it connects up.
It is from these connected knowledge linkages we can build a learning memory and extract expertise to become the basis of our collective intelligence. We can discover, learn and build from this ‘connecting all our knowledge up’ to see new possibilities, and that is where Knowledge graphs offer, for me, the most exciting possibilities for innovation, a new discovery.
They can dramatically improve our domain knowledge, improve our common sense and discovery, by linking the relevant context and through this decent labeling of this, we can then manage and interpret far more complex schemas. Up to now knowledge has been kept “closed down” and used fairly inefficiently, parceled out sparingly, so it more than often constrains greater understanding. We have allowed it to form, ossify and become rigid and static, of limited use. It struggles to be reused or recombined into new insights, as we lack the ability to access islands of knowledge and form the relationships.
Knowledge calls for a constant re-working so we can be re-applied in a new context. It is knowledge coupled with insights that lead to new innovation. Knowledge Graphs sound tantalizing, will they be the next big thing?
- In my research and reviews on Knowledge Graphs, I found some useful references. Specifically IDC in some of their white papers on Semantic Technologies to give a deeper background understanding
Another variety of knowledge graphs — we might call them, “knowledge maps” — are the perp boards used by law enforcement and investigative agencies, popularized on almost every single spy or police drama we’ve seen on TV and in the movies. Various items of interest are tacked up and strings used to associate them. Currently, because it doesn’t matter on TV or on film, they’re all 2D. Using visualization technology, they can now be produced in 3D, and their constituents more easily manipulated. Unlike knowledge graphs — at least as KGs are currently represented — that visually standardize data, knowledge maps seem limitless in terms of the variety of types of data they can display and work on, albeit often as approximations or simulations of real objects. It would be interesting to produce a comprehensive collection of exemplars of the various types of graphing and mapping techniques as applied to knowledge graphing and mapping, with a critical appraisal of their utility in different situations and for an array of purposes. Has this already been done? If not, what would it take to produce such an encyclopedia of types and functions?
PS This is very interesting stuff! As might be expected from an innovation guru who’s learned to sift the grain from the chaff!
Certainly appreciate that compliment Bob- thank you!
A good question Bob. Firstly thank you for your contribution. I feel maps and graphs is fairly lose for much, establishing their value and utility would be highly useful. We need someone to put their hand up and invest time in this. What it takes, to be honest I have no clue, I am sure there is someone out there completing some of this work, maybe google themselves
what a timely and relevant topic Paul and really well written. Thank you.
Our company is currently in the midst of introducing to a major auto brand what kind of infrastructure can be developed (from an existing silo of highly redundant information) and how that infrastructure can improve the data indexing and retrieval within the organization, the retrieval improvement is invaluable alone. This now allows the company to connect to the front end for marketing purposes, unheard of.
Most think the keyword really is expediency. Few companies realize the value of resources saved with semantic data catalogs, and without correctly implemented structured data markup they’ll miss out on voice search (phone, digital assistants, car audio), AI, mobile search, and smart contract interaction. Better to start the infrastructure now, its no longer a question of necessity.
Thanks for the post.